RECLAIM is a portable material recovery facility packed in a box but we are actually thinking outside of it!
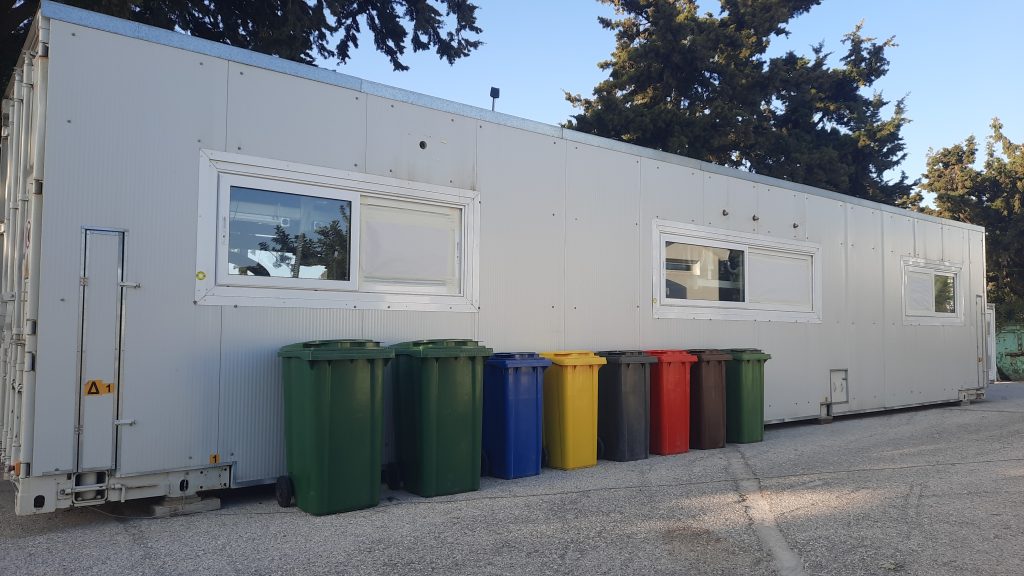
The last 18 months have been an intense test-bed of expending energy on experimentation and implementation of our solution at RECLAIM project. While we made good use of robotics, grippers, advanced imaging and artificial intelligence to get closer to our objectives, excellent human connections and synergies within the consortium drove this innovation. Let’s just say this is a good work-in-progress of the marriage between humans and robots. More is yet to come!
Now, let us cut to the chase, and understand what’s inside the box!
Objective 1: Design and implement an eco-friendly prMRF
Progress Achieved: In the first 18 months, RECLAIM project has successfully installed all the equipment involved in the monitoring, categorisation and physical sorting of recyclables within the prMRF. Thus, the prMRF is fully functional and can perform all planned tasks.
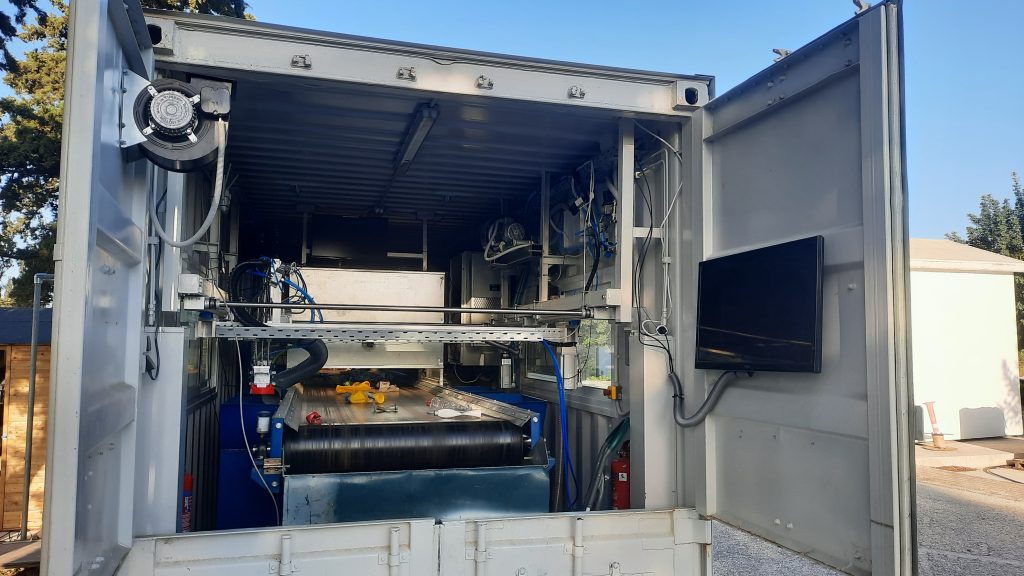
How was this achieved?
The prMRF was temporarily set up at the FORTH premises for integration and testing of its modules. This allowed evaluation of its performance using realistic waste materials but not with the actual “raw” content of the Blue-bin (Plastic, Metal, Drink packaging), as this would require additional mechanical equipment for the pre- and post-processing of the waste. The good news though is that HERRCO has already tested the pre-and post processing waste equipment provided by ION is readily available for use in Kefalonia for the planned pilot tests.
Thanks to the complementary expertise of, on one hand, HERRCO, in large scale MRF design implementation and operations, and on the other, ROBENSO, in waste sorting automation using robots. With their support, further project developments are now on aligned and on track.
Future work: Going ahead, further improvements are needed with respect to targeted performance, for instance, increasing the successful picks/minute rate.
Objective 2: Improve robotic recyclable recovery systems
Progress achieved: The project developed, implemented and evaluated the performance of a simplified, low-cost robotic solution called Robotic Recycling Worker (RoReWo), specifically designed for waste sorting. This innovative approach uses a piston mechanism to swiftly move a vacuum gripper over waste, minimising the need for intricate handling of compacted and deformed waste packages. The RoReWo stands out for its substantially lower implementation costs, sacrificing only a modest percentage of performance.
Installation of the 1.5 DoF RoReWos inside the prMRF
In addition, the project has explored different gripper technologies based on ease of integration and applicability in different RoReWo version in order to improve waste sorting efficiency. For example, the project explored multiple gripper features: the availability of grasp planning algorithms, the compatibility with cluttered environments, the compatibility with the characteristics of the waste materials that need to be grasped, speed with which the grasping technique is able to grasp and release the waste materials, the safety aspect of the grasping technique and how many degrees of freedom are required to use the specific gripper techniques for the sorting of waste materials.
KU Leuven provided three gripper designs — parallel grippers with conventional and soft fingers, and the HAVCO gripper—fitted on the 2.5 and 3.0 DoF RoReWos, while custom-designed vacuum grippers by Robenso were outfitted on the remaining 1.5DoF RoReWos. Three grippers have been deployed and evaluated for effectiveness across various material types and shapes within the prMRF.
The mounting of different grippers on RoReWos before being tested inside the prMRF.
In a clear message to industry and developers, the project has used multiple RoReWos inside a single prMRF by deploying multiple-robots/multiple gripper approach to increase waste picking robustness and overall prMRF productivity.
Future work: The final choice of gripper will be determined based on their effectiveness and the material composition in the incoming waste stream.
Objective 3: Advance and exploit state of the art AI technologies to effectively tackle the identification, categorisation and localisation of recyclable waste
Progress achieved: In the reporting period, the project was better able to identify, localise and categorise wastewith the help of AI, Deep Learning (DL) and advanced imaging technologies. In concrete terms, this means higher quality and quantity of recyclable materials collected from mixed waste streams, which will benefit the industry, developers and scientific communities.
How was this achieved?
After gathering and annotating, ie., labelling or marking, RGB images for machine learning, the project introduced a new annotation tool integrating instance segmentation that distinguishes between similar but overlapping objects allowing to accelerate labelling of waste images captured within the prRMF.
The above figure shows the HSI chemometric models in action, making material predictions for different objects.
But how does this neural network function in practice?
This component checks how realistic the synthetic images (computer generated images that mimic real-world images) look. By generating many artificial single-object images and keeping only the most realistic ones, the project improved the training of Mask R-CNN, thereby ensuring accurate and efficient waste detection and sorting. Industries, developers, and scientific communities can greatly benefit from using AI, deep learning and advanced imaging solutions.
Future work: In the following period, the project will fine-tune the combination of the hyperspectral and the RGB-based waste recognition modules in order to recognise over 95% of the treatable recyclables from the mixed waste stream. Recyclable waste databases will continue to be populated along with annotated hyperspectral and RGB images.
Objective 4: Deploy prMRF to realise the recovery and sorting of recyclables in targeted areas
Progress achieved: The project has been able to successfully evaluate the integrated performance of the fully assembled prMRF in realistic conditions that resemble its intended working environment and get valuable feedback on the integrated operation of its components.
Initially, procedural difficulties and integration issues regarding fitting the mechanical equipment alongside computer vision, Hyperspectral Imaging (HSI) and robotic hardware inside the constrained prMRF space delayed the operational process. However, with the technical support of partners, small adjustments were made to complete the implementation of the prMRF before the first half of the project itself, which is a significant achievement.
It is expected that the prMRF will be deployed and will become operational all within a single day. The precise time required for deployment will be considered during the prMRF’s relocation to Kefalonia island.
Future work: In the second half of the project, the project will carry out investigations into the allocation of roles for RoReWo based on waste distribution. Initial experiments show that RoReWos can effectively handle recyclables in various deformed shapes. However, more experiments at the actual deployment sites are needed to verify this key performance indicator. Additionally, practical experiments need to be conducted in order to determine the performance of the prMRF, supported by periodic AI-ILC re-training, to successfully treat over 50% of miscategorised recyclables.
Objective 5: Increase social sensitivity and engage citizens in material recovery research
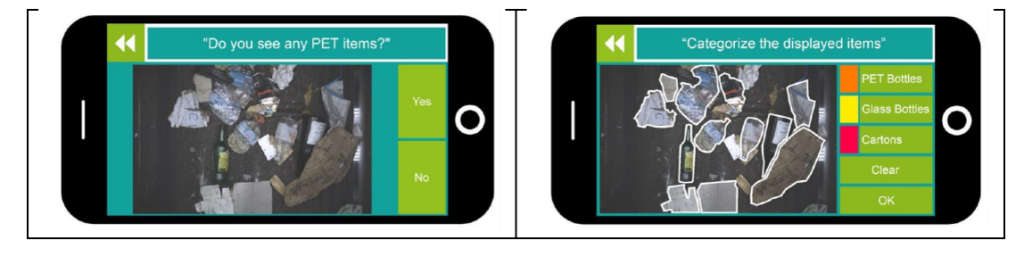
Progress achieved: Following data collection using human annotation of waste for engagement in material recovery research,the University of Malta developed a knowledge base of facts and questions supported by discussions with circular economy and dissemination experts, HERRCO and ISWA. Aim is to enhance curiosity and awareness across seven recycling-related topics. Presently, the project is evaluating human annotation challenges through 10 different mini-games with stakeholders who also contributed to requirements collection for the game.
Utilising human inputs, the RDG will be able to better train the Artificial Intelligence models using real-world data, in turn refining recycling automation technologies. This aspect is useful, especially for the general public, policymakers, developers and scientific communities.
The above image shows the final setup for waste data collection as part of the functional prMRF
Future Work: The RDG game is still under development and has only been tested with focus groups of a few users. Before the game is made available to the broad public, additional development efforts are needed (especially to raise awareness of recycling) and is targeted to occur in the second half of the project. Future work will involve focus group discussions to gauge the effectiveness of these facts and questions in raising awareness and adjust accordingly to maximise the impact of the RDG on awareness.
In the game design phase, the project developed over 100 recycling facts along with corresponding question and answer sets to boost curiosity (via the question) and awareness (through the subsequent fact). However, this content has not yet been integrated into the recycling data game and is slated for inclusion in the next iteration.
Objective 6: Maximise project Innovation impact
Progress achieved: Significant effort has gone into crafting the prMRF sustainability plan, ensuring its operation for at least 5 years post-project. This period focused on identifying and estimating key revenue streams, conducting CAPEX-OPEX calculations, defining the break-even point, and determining ROI. Results indicate a strong potential for prMRF sustainability.
Future work: The business models for RoReWos and AI-ILC will be developed in the project’s latter half. In addition, the project has identified and contacted five “sister projects” from Horizon Europe Clusters, and by month 18, connected with the Grinner Project, exchanging basic project data and objectives. Collaboration is also underway with the SOPRANO project and the AI-on-demand (AIOD) platform, where RECLAIM has published multiple articles.